Learning is not a process of accumulation of representations of the environment; it is a continuous process of transformation of behavior through continuous change in the capacity of the nervous system to synthesize it.
—Humberto R. Maturana1
The short film “Tony de Peltrie” debuted in 1985, at the 12th SIGGRAPH conference on computer graphics in San Francisco. Created by a team of programmers at University of Montréal, the film’s digital lead character may look goofy and pixelated today, but at its premier the audience was stunned by its realism. Time magazine praised the film as a landmark in simulation: “De Peltrie looks and acts human; his fingers and facial expressions are soft, lifelike and wonderfully appealing. In creating De Peltrie, the Montreal team may have achieved a breakthrough: a digitized character with whom a human audience can identify.”2 The film was the first time in cinematic history that facial animation played a major part in conveying a story. In doing so, it not only ushered in a new age of ever-more lifelike face animations, but also techniques to automate facial expression detection. Technological observation and reproduction are two sides of the same coin: they both break down the anatomical face into computable parts to decipher or simulate its signals. In order to do this, a set of predefined “emotion identifiers” are necessary; categories such as “happiness,” “love,” “joy,” and “anger.” Such categories cluster emotions into generalized expressions that are assumed to be physically visible through body language. Omitting their subjective experience, silent sensation, and cultural influence, emotions need to be compressed into universal signals to be useful in the world of computation.
All The Feels
In 1981 at the Department of Computer and Information Science of the University of Pennsylvania, Stephen Platt and Norman Badler investigated the development of a “total human movement simulator” to perform American Sign Language.3 While engaged in creating a digital character and animating its body, they realized that facial expressions would play a crucial part in non-verbal communication.4 They describe their early face simulation attempts as “[encoding] actions performable on the face.” Platt and Badler found that one of the key aspects in creating believable expressions was to identify areas of the face to animate. They introduced an algorithm that simulated muscle contraction and coordinated a series of movements to result in a facial expression. In order to simulate “doubt,” for example, a series of animations were triggered throughout the face: a raised left eyebrow, slightly wrinkled nostrils. These triggered areas represent a low-resolution simplification of facial anatomy. Rather than defining these areas themselves, however, Platt and Badler were first to apply the Facial Action Coding System (FACS) to a mesh face model.
Developed as a system to describe facial expressions for psychological analysis by psychologist Paul Ekman and Wallace Friesen in 1978, the Facial Action Coding System is an index of visually detectable facial expressions. FACS’s classification of muscle movement into numbered Action Units (AU) is based on earlier work by anatomist Carl-Herman Hjorstjö.5 Still, FACS is not the first system to propose a universal categorization of emotions: Charles Darwin published a similar theory in 1872 introducing the hypothesis that facial expressions present emotions regardless of species, not to mention culture. Darwin’s study built on the long-disputed pseudoscience of physiognomy that demonstrated a connection between a person’s appearance and character. What distinguishes FACS from previous work is that it pushed this belief of universal observation into a measurable system and quantifiable future. Its publication coincided with the first Computer Generated Imagery (CGI) experiments of animating a face by Frederik Parke in 1972, and the subsequent application of FACS towards more realistic expressions by Platt and Badler. FACS was thus appropriately introduced at a time when the computer graphics industry was starting to grapple with the complexities of depicting human life, presenting a neatly packaged solution to replicate manifold complexity on screen.
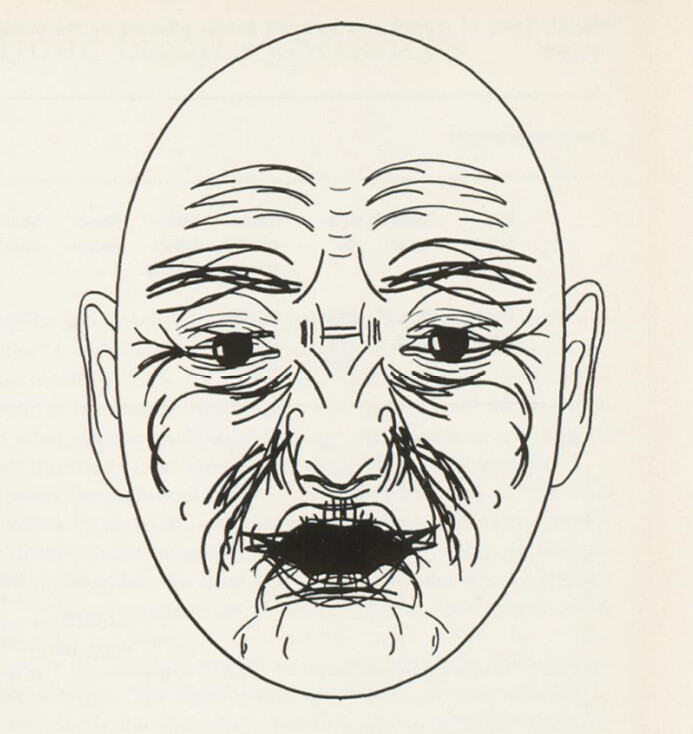
Schematic presentation in a single picture of all muscle effects. Carl-Herman Hjortsjö, Man’s Face and Mimic Language (1970), 65.
Polygonal Crocodile Tears
The field of CGI obsessively strives towards real-time high-resolution visuals. Rendered imagery permeates many areas of contemporary life, at times undetected, from the movie industry, to video games, to ergonomic simulation software, architectural visualizations, AI-enhanced mobile phone cameras, animated emoji’s, and news agencies. The manic recreation of skin pores, subsurface blood vessels, and tear light refractions in evinces that simulating reality to the point of deception is recognized as a benchmark to computer graphics’ capabilities. Referred to as “photorealistic CGI,” the term neglects critical discussions in photographic discourse on representation, truth, and framing. Ironically, in CGI, “photorealism” refers to images that successfully deceive the viewer. These types of visuals are certainly not the only kind that can be produced with CGI. On the contrary: the technology invites for reality to be surpassed. However, an effective photorealistic image most effectively speaks to the technical sophistication of the software, the author’s craft, and their hardware resources. It is a muscle flex, and a receipt of the available production budget.
Within the practice of photorealistic CGI, synthetic, animated human bodies and faces are the ultimate capability measure. Humans instinctively detect when features of a synthetic human are off. The concept of the “uncanny valley” was coined by robotics researcher Masahari Mori in 1970 to describe the eerie sensation of encountering an almost-but-not-quite-realistic humanoid robot. The same concept is used to describe nearly “photorealistic” synthetic humans on screen. An infamous example is 2004’s animation feature film Polar Express, in which actor Tom Hanks played several characters, from a boy to a conductor, with the help of performance capture technology. Hanks’s acting was recorded in an almost-empty space, save for infrared cameras recording the movement of several white dots placed on a Spandex suit he was wearing. In post-production, this captured performance was then transferred to the various digital characters he was to embody on screen. The face animations in Polar Express were particularly haunting. One reviewer complimented the character’s facial expressions during “extreme emotions” but otherwise felt the characters were lifeless: “you can knock, but nobody’s home.”6 Polar Express fell into the visual gap between caricature animation and photorealistic imitation that left viewers uneasy.
Moving forward in time to Martin Scorsese’s 2019 three-hour epic The Irishman, facial animation has taken another leap. The film’s script had been written years in advance, but its production depended on technology to be developed to virtually age and de-age the actors throughout the movie’s fifty-year timeline. Not only was the technology expected to shapeshift actors across time, it also had to be done without wearing any performance capture suits or calibration marker make-up. Scorsese wanted the technology to be invisible on set. Next to a special three-camera rig that recorded infrared and color imagery, the actors’ heads were 3D-scanned by a system called Medusa. Developed by Disney Research and now in the hands of Industrial Light and Magic, Medusa captures “high-resolution 3D faces, with the ability to track individual pores and wrinkles over time, and can recover per-frame dynamic appearance (blood flow and shininess of the skin), providing a very realistic virtual face that is ideal for creating digital doubles for visual effects and computer games.”7 Notably, Medusa is used to create an expression shape library of each principal actor by recording “FACS expressions” that then can be blended and mixed in post-production.8
The future dreams of a digital production workflow that can take a recorded performance and train an artificial intelligence in imitating and elaborating said performance. Recent experiments in applying AI technology to video footage include deepfakes. However, rather than using a dataset to train a predictive model that can surpass the source material, deepfakes twist the content of existing footage by replacing a face or retargeting lip sync patterns. A digital character could move through a virtual world autonomously, react to input down to the last blood and tear drop of emotional detail. As The Irishman showcases, actor’s real-life age and appearance ceases to be all that important. Perhaps digital actors can live longer and act in movies after their physical bodies have passed away. This speaks of yet another paradox of “photorealistic CGI”: once there is no reference, the actor has passed, what realism is it then producing? Does it surpass the living by deceiving? In the age of artificial intelligence, computer vision, and virtual cameras, training data of “real world” situations becomes ever more important. In such an environment, The Irishman actor Robert De Niro’s expression shape library is extremely valuable, as it can be used to train a neural network to act on it’s own, while imitating De Niro. A quote from a recent paper describing a 3D face library for artificial intelligence training describes the role of FACS in this development: “it provides a good compromise between realism and control, and adequately describes most expressions of the human face.”9 The question then remains, is adequate realism the kind we want to inhabit?
A Different Kind of Performance Capture
Beyond its adoption in computer animation, FACS remains “relevant” as a psychological tool today in everything from police profiling to health assessments, career placement, and anthropology. The wide variety of use cases speaks to the universality of its system, and enables yet another intersection of FACS and computation: the automation of emotion recognition. Deployed as a machinic backup in circumstances where a human is not able to register minute changes in facial expressions, like in a crowded space or a multitasking situation such as a job interview, the automation of emotion recognition promises detection through a combination of computer vision and machine learning.
While FACS does not offer interpretation of facial expressions as emotions, it’s indexing system serves as foundation and framework. Ekman refers to these difficult to spot, brisk changes in features as “micro expressions”: “facial expressions that occur within a fraction of a second.”10 What this technology promises to achieve is recognition of anomalies at capture speeds impossible to humans. Importantly, emotional recognition technology does not “read” what it “sees” better than a trained individual, but it’s ability to pick out a detail that is invisible to the human eye might make it seem more capable. Invariably, the interpretation of the detected signal involves human judgment, and thus inherent prejudice. Signal analysis is either performed by personnel or, depending on the method, automated by training a system according to a predefined dataset of emotional cues. These datasets can be assembled from two types of information: either actors being recorded performing various Action Units or video clips collected “in the wild” that are then categorized according to the FACS facial expression index.11
Following the events of 9/11, Ekman argued that the attacks on New York’s World Trade Center could have been prevented if airports were outfitted with emotion-reading machines, to reveal potential malicious intentions.12 He has since worked with the CIA, the US Department of Defense, and the US Department of Homeland Security to train personnel and develop machines for exactly this application. In 2007, the Transportation Security Administration (TSA) has introduced a new screening protocol at US airports called SPOT, an acronym for “Screening of Passengers by Observation Techniques.”13 SPOT trains “Behavior Detection Officers” based on a design by Ekman to detect “the fear of being discovered.”14 The program has received ample critique as conducting racist profiling and being bogus science.15 The US Government Accountability Office even filed a research report on SPOT in 2013 stating that there was no evidence that “behavioral indicators … can be used to identify persons who may pose a risk to aviation security.”16 TSA has since defended the accuracy of SPOT, and funded a research into micro expressions’ ability to act as detector of malicious intent in 2018.17
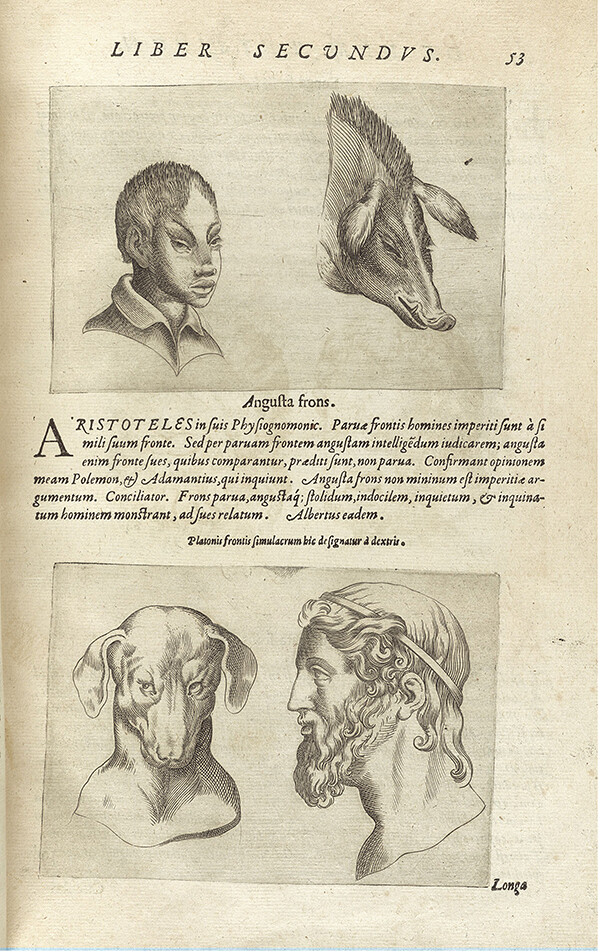
Giambattista della Porta, De Humana Physiognomonia libri IIII (1586).
These applications follow the belief that the face holds the key to truth and reveals thoughts left unspoken. Ekman refers to micro expressions as an “involuntary emotional leakage [that] exposes a person’s true emotions.”18 In the case of airport screenings, automated facial expression recognition takes on a predictive function by claiming to pick up signals of future intentions. Such expectation echoes the practice of physiognomy, a pseudoscience that originated in antiquity and resurged in Europe during the late Middle Ages to assess a persons character from their outer appearance. Several studies at the time illustrated the hypothesized connection between the appearance and character traits ascribed to animals and humans,19 while disguising prejudice in scientific diagrams.20 Others interpreted facial features as signs of intelligence and ability.21
Physiognomy has long-since been dismissed as ungrounded practice, but is experiencing a new renaissance with the need for categorization and classification to formulate machine learning algorithms. One particularly troubling recent example was the 2017 research paper by Yilun Wang and Michael Kosinski which announced to have built an AI-powered “gaydar” capable of detecting sexual orientation based on people’s faces.22 The training data and applied categories are particularly important to scrutinize in this research, as its coded rationality can bear harmful consequences affecting real lives. Furthermore, the research takes for granted that sexual orientation is indeed to be divided up into categories, rather than being a spectrum that encompasses more attributes than might be visible in a person’s photograph or their presenting gender.
At first glance, the automation of emotion detection can appear trivial compared to Wang and Kosinki’s research. However, as becomes apparent in cases where it is used to profile possible criminal intent, such as at US airports, the perception of a technology quickly changes depending on it’s application. Each newly developed technology should be subject to the same kind of scrutiny as any other, precisely because it’s end application is often unclear at the moment of it’s development. Yet any single technology, such as automated emotion detection, is applied in multiple, varying scenarios, each with their own set of potential consequences.
Affectiva, one of the world’s leading “emotion measurement” companies, has been using FACS to recognize and label facial expressions that map to six core emotions: disgust, fear, joy, surprise, anger, and sadness. According to Affectiva, these core emotions are universally recognized and defined by Ekman’’s studies. The interpretation of facial expressions as emotions is where value is created: the company partners with various areassectors, such as the automotive industry to monitor driver attention and passenger experiences, or media agencies in analyzing advertising reception. FACS is as useful as it is problematic. In early computer animation research, the system of facial expressions was used as a practical solution to advance technology. Subsequent work was built upon and referencing these achievements, internalizing FACS along the way. Gradually, FACS became the standard tool to scrutinize the human face, trickling from academic research to real world application. It’s claim to universal applicability makes it the perfect foundation for building globally marketable products.
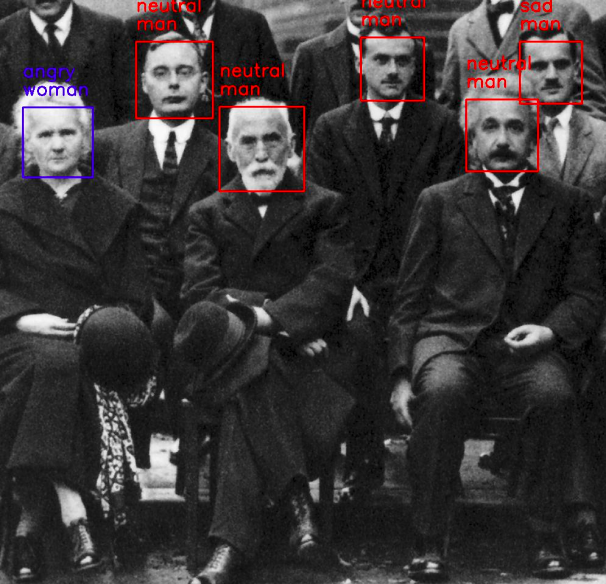
Group Photo of the 1927 Solvay Conference on Quantum Mechanics as seen through an emotion and gender identifying neural network. Marie Curie, the only attending woman, is the sole face labelled “angry.” From Octavio Arriaga, Matias Valdenegro-Toro, and Paul Plöger, Real-time Convolutional Neural Networks for Emotion and Gender Classification (2017).
Behavior Management
Emotion measurement software, as it is developed by Affectiva and similar companies, is not dependent on new hardware. Recognition software can analyze footage captured by any camera, airport CCTV, laptop webcam, or dashboard camera. In fact, this type of technology relies on large amounts of pre-existing footage to be able to train their system in recognizing emotions. The independence from hardware is significant in that the technology’’s use does not come with a physical signifier, a new machine. The ubiquity of cameras in contemporary life allows for an already distributed multitude of applications.
One area that has recently introduced such technological assistance is the job recruitment process. Employment interviews are increasingly conducted remotely via cameras through video call or self-recorded clips, in which candidates answer a catalogue of questions provided by the prospective employer. Video interviews are then subjected to an emotion analysis to evaluate the applicants on predefined criteria. Unilever is among recent adopters of the technology and lists candidates on a scale of one to hundred ”against the database of traits of previous ‘successful’ candidates.”23
Emotion analysis is marketed to employers as fast, neutral alternative that gets the position filled within a fraction of the time it takes human recruiters. It’s claim for neutrality, over the bias of a human recruiter, stands to be challenged.24 25 Recognizing emotion is often enough already challenging for humans. Did this person smile out of courtesy, joy, or pity? Employing automation will not ease the burden of interpretation or offer further insight. A camera might record at a staggering resolution, and the algorithm might be trained on a “very diverse and vast” dataset, but the translation of facial expressions to emotions is still performed and informed by human subjectivity and will inherently contain bias. The ambition to create a global, general applicable product might makes sense in terms of marketing, but it is a trap for discrimination, especially when encoded into automated systems.
Job interviews are already notoriously stressful and uncomfortable. A faceless metric as a counterpart certainly does not soothe the nerves. Various YouTube tutorials offer advice on how to present oneself to this new machinic recruiter. There is plenty of advice given to “just be you.” In Harun Farocki’s 1997 documentary film Die Bewerbung, the viewer follows different people as they attend a job interview training workshop.26 Watching these students practicing the interview process and training how to present themselves, awkwardly underlines the performative aspect of the application process. Perhaps an updated training workshop is in order, a behavior management course in self-representation towards cameras. How to act “natural” while also performing facial expressions that register positively? How to succeed in an interview when the conversation is not a negotiation but a one-way interrogation?
Humberto R. Maturana, Biology of Cognition, 1970, Reprinted in Humberto R. Maturana and Francisco Varela, Autopoiesis and Cognition: The Realization of the Living (Dordrecht: Reidel, 1980).
Phillip Elmer-DeWitt, Time Magazine August 5, 1985.
Norman Badler also conducted research on the first digital human figure known as JACK. Developed for animation purposes the avatar has been used in ergonomic design software to test human centered environments and objects before they go into production. The development and use of JACK comes with a set of critical questions on the necessity to standardize the human for computation and the resulting exclusion of bodies. For more on this, see Simone C. Niquille, “SimFactory,” Artificial Labor (e-flux Architecture, September 22, 2017), ➝.
Stephen Platt and Norman Badler, “Animating Facial Expressions,” ACM SIGGRAPH Computer Graphics (1981).
Carl-Herman Hjortsjö, Man’s face and mimic language. (Lund: Studen Litteratur, 1970).
Paul Clinton, “Review: ‘Polar Express’ a creepy ride,” CNN.com, November 10, 2004, ➝.
➝.
Netflix, “How The Irishman’s Groundbreaking VFX Took Anti-Aging To the Next Level,” YouTube, January 4, 2020, ➝.
Cao Chen, Yanlin Weng, Shun Zhou, Yiying Tong, and Kun Zhou, “FaceWarehouse: a 3D Facial Expression Database for Visual Computing,” IEEE Transactions on Visualization and Computer Graphics 20, no. 3 (2014): 413–425.
Paul Ekman Group, “Micro Expressions,” ➝.
The term “in the wild” refers to machine learning data that has not been recorded for the research purpose at hand but rather has been collected from other sources to ensure “authenticity.” Publicly accessible sites such as the photo sharing website Flickr or the video platform YouTube are frequented repositories as are corporate datasets such as Uber user data or iRobot’s vacuum cleaner’s indoor maps of clients homes.
Paul Ekman, “How to Spot a Terrorist on the Fly,” Washington Post, October 29, 2006, ➝.
Sharon Weinberger, “Airport security: Intent to deceive?,” Nature 465 (2010): 412–415, ➝.
Nate Anderson, “TSA’s got 94 signs to ID terrorists, but they’re unproven by science,” Ars Technica, November 14, 2013, ➝.
“The human face very obviously displays emotion,” says Maria Hartwig, a psychology professor at the City University of New York’s John Jay College of Criminal Justice. But linking those displays to deception is “a leap of gargantuan dimensions not supported by scientific evidence.” See Weinberger, “Airport security,” ➝. The American Civil Liberties Union has filed a Freedom of Information Act lawsuit against TSA in 2015 to obtain documents on SPOT’s effectiveness. Hugh Handeyside, staff attorney with the ACLU National Security Project. “The TSA has insisted on keeping documents about SPOT secret, but the agency can’t hide the fact that there’s no evidence the program works. The discriminatory racial profiling that SPOT has apparently led to only reinforces that the public needs to know more about how this program is used and with what consequences for Americans’ rights.” See ACLU, “TSA refusing to release documents about spot program’s effectiveness, racial profiling impact,” March 19, 2015, ➝.
US Government Accountability Office, “TSA Should Limit Future Funding for Behavior Detection Activities,” November 8, 2013, ➝.
David Matsumoto and Hyisung C. Hwang, “Microexpressions Differentiate Truths From Lies About Future Malicious Intent,” Frontiers in Psychology, December 18, 2018, ➝.
Paul Ekman Group, “Micro Expressions,” ➝.
Giambattista della Porta, De Humana Physiognomonia libri IIII (1586), ➝.
Ibid.
Johann Kaspar Lavater, Physiognomische Fragmente zur Beförderung der Menschenkenntnis und Menschenliebe (Verlag Heinrich Steiners und Compagnie, 1783), ➝.
Yilun Wang and Michal Kosinski, “Deep Neural Networks Are More Accurate than Humans at Detecting Sexual Orientation from Facial Images,” Journal of Personality and Social Psychology 114, no. 2 (February 2018): 246–257.
Charles Hymas, “AI used for first time in job interviews in UK to find best applicants,” The Telegraph, September 27, 2019, ➝.
Yi Xu, founder and chief executive of Human, another company offering AI emotion measurement services, said: “An interviewer will have bias, but [with technology
they don’t judge the face but the personality of the applicant.” See “How AI helps recruiters track jobseekers’ emotions,” Financial Times, February 2018, ➝. Loren Larsen, Chief Technology Officer at HireVue, when asked about Unilever using their technology, said: “I would much prefer having my first screening with an algorithm that treats me fairly rather than one that depends on how tired the recruiter is that day.” Hymas, “AI used for first time,” ➝.
Farun Farocki, Die Bewerbung, 1997, ➝.
Intelligence is an Online ↔ Offline collaboration between e-flux Architecture and BIO26| Common Knowledge, the 26th Biennial of Design Ljubljana, Slovenia.