To make machines look intelligent it was necessary that the sources of their power, the labor force which surrounded and ran them, be rendered invisible.
—Simon SchafferIf a machine is expected to be infallible, it cannot also be intelligent.
—Alan Turing
Metacognition in the Twenty-First Century California Adult
The idea that “machines think” displays an unintended solidarity with the animism of less industrialized cultures, which have long recognized autonomous minds in nonhuman entities. Artificial intelligence is animism for the rich, we might say. Or alternatively: animism is a sort of artificial intelligence made in the absence of electricity.1 The recent narrative which proclaims the imminent arrival of a Technological Singularity (according to which computing machines would become self-conscious) seems typical of the human tendency to anthropomorphize the unknown. What was once attributed to the obscure and infinite night is now projected onto the abstract abyss of computation, data centers, and machine learning. Rendering the uncanny (Unheimlich) familiar (heimlich) by way of mythology is an established survival method for the human animal in the act of mapping its territory. In a hostile environment, the utility of suspicion towards any alien object is obvious: even if it doesn’t appear to move, it may be alive and dangerous. The same seems to be true even for the most advanced technological milieu. In psychology, this ability to speculate that other beings might have a will, drives, or “thoughts” less friendly than what they articulate is called metacognition, or Theory of Mind (ToM).
The Theory of Mind is a key issue in child psychology. As infants we do not know what our mother thinks: the first relation with her is a blind metabolic one, a need for milk, warmth, and care. Only gradually do we develop the understanding that our mother does not always fulfill our desires, that she may have different intentions and thoughts than ours. That is the traumatic moment in which we project the theater of the mind, i.e., we “theorize” the mind into another body. However, Soviet psychologist Lev Vygotsky stressed that we form the image of our mind only after picturing the mind of adults around us. Growing older, we develop an even more sophisticated mind reading: in playing poker or listening to a politician speaking, we always engage in simulating the backstage, imagining the other’s mind tricks behind and beyond their appearance. This process may unfold into pathological excess, like in the case of paranoia and conspiracy theories, when an unreachable evil mind is evoked to explain catastrophes too big to be elaborated. Or we may prefer to project a mind onto the furnace below the window or develop a vast objectophilia like Eija-Riitta Eklöf, who married the Berlin Wall.
As art critic and curator Anselm Franke suggests, animism is a good epistemic prism for capturing the many refractions and responses to industrialized modernity. Artificial intelligence inevitably belongs to this history: “Animism had endowed things with souls; industrialism makes souls into things.”2 British computer scientist Stephen Wolfram has argued that the universe is fundamentally digital in nature, and that natural laws are better approached as computational programs rather than instances of traditional mathematics. Wolfram argues that animism somehow is an acknowledgement of nature’s computational power: a doctrine that can be called “computational animism.” This latest animism turns panpsychism, the idea that everything thinks, into pancomputationalism, the idea that everything computes (especially for business purposes).3
An abnormal Theory of Mind is common to all ages and classes, usually as a substitute for confronting more fundamental political issues. It is not surprising that the ruling-class engineers of California have started to anthropomorphize supercomputers and fear their awakening as sentient and autonomous beings, while Silicon Valley entrepreneur Elon Musk has warned against the risk posed by future machine intelligence.4 In a clinical sense, the narrative of Singularity is a good example of the faculty of metacognition in the twenty-first century Californian adult, as surely as the Theory of Mind explains many popular publications on so-called “artificial superintelligence.” Time to ask Western anthropologists to visit the valleys of Northern California.
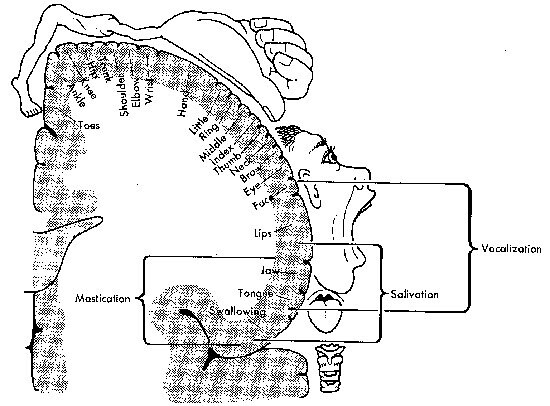
Two Lineages of Machine Intelligence
The history of machine intelligence can be roughly divided into two lineages: the analytical one, based on the idea of the representative brain, and the holistic one, based on the idea of the adaptive brain.5 Where the analytical emphasizes logic, the holistic emphasizes abstraction, considering the human brain as an organism that strives to adapt to the surrounding world and which conceives and projects new ideas (Gestalten) in the course of this process. In the holistic, adaptive tradition, intelligence is understood as an antagonistic and embodied relation with the environment. In fact, the idea of the cybernetic feedback loop was inspired by this model of biological adaptation.6 This is the lineage of Norbert Wiener, William Ross Ashby, and Anglo-American cybernetics influenced by German Naturphilosophie. Epistemologist David Bates has noticed that early cybernetics, being particularly influenced by neurology and cognitive sciences, was not just obsessed with the mechanization of reason but interested also in the abnormal states of machines, in those pathological breakdowns that could push machine structure to form new equilibria and new compositions.7
The epistemic distinction between the two lineages is the distinction between analytic logic and perceptual Gestalt as universal diagrams of human thought. They are not a perfect contrast: perceptual Gestalten, for example, would be encoded into information by Wiener while digital logic would become purely statistical and “gestaltic”in the process of encoding chaotic phenomena. In his founding text Cybernetics (1948), Wiener wrote that cybernetics would more closely resemble a predictive and statistical discipline such as meteorology than it would the exact laws of physics. And one could do worse for a description of the study of the human brain than a “meteorology of intelligence.”8
The analytical lineage, as mentioned, sees the human brain as a machine that represents the world through language. Specifically, it states that human thought can be translated by Boolean logic into logic gates, the elementary building blocks that construct digital circuits, transistors, and microchips out of three fundamental functions: AND, OR, and NOT. The mind can be mechanized thanks to this equivalence between human logic, Boolean logic, and logic gates. This is the legacy of Gottfried Leibniz, Charles Babbage, and Alan Turing. Turing is famous for introducing the Universal Turing Machine, the abstract algorithm that separated for the first time software and hardware in computation. But he also contributed to the history of philosophy with a controversial intervention into the Theory of Mind: the Turing Test.
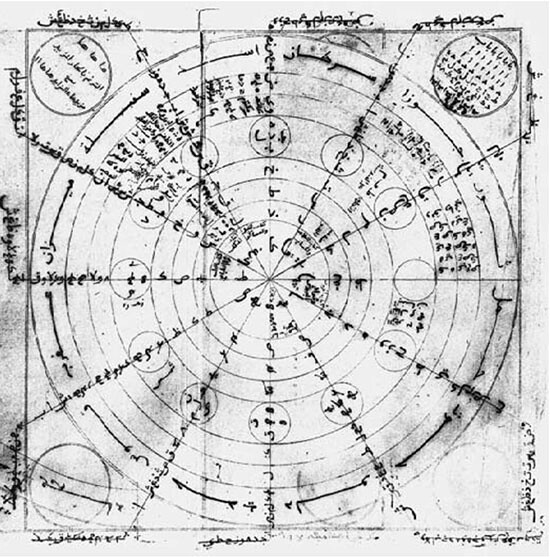
Turing’s Foray into the Theory of Mind
In his 1950 paper “Computing Machinery and Intelligence,” Turing aimed to resolve the question: “Can machines think?” He proposed to do this via a negative thought experiment, the Turing Test, also known as the Imitation Game. Rather than asking what the positive content of intelligent behavior must be, Turing reasoned that if we humans cannot tell the difference between the answers given by a machine and a human—when these are disguised by a textual interface—then we cannot say that machines do not think. With its absolute emphasis on the social convention of human language, the Turing Test is the ultimate Gedankenexperiment of Analytical Philosophy and a metaphysical reiteration of the Theory of Mind. The test reinforces, rather than questions, the metacognitive assumptions behind artificial intelligence, precisely by advancing computation as empirical proof of thought in nonhuman entities. The Turing Test does not prove machine intelligence per se, but it postulates that an anthropomorphic Theory of Mind can be logically imbricated in computation. Moreover, it does not just imply that artificial intelligence has to pedantically resemble the human—a crystalline example of the anthropomorphic fallacy—but it renders artificial intelligence as brute force imitation of human habits and conventions, a grand machine for the recognition of the Same.
By employing a schema of mind that prioritizes good manners and familiarity with social conventions, the Turing Test remains an example of austere social normativity: the same one, it has been suggested, that Turing himself was subjected to as a closeted gay man. Turing addresses briefly the issue of metacognition in the answers to the “Arguments from Various Disabilities,” where he mentions the fact that a machine could “be the subject of its own thought” by running a program to check its own structure, again rendering the Theory of Mind in terms of computation.
In the history of the systemic and holistic thought, a famous counterpart to the Turing Test is Hegel’s master-slave dialectic, which also attempts to explain self-consciousness via the recognition of consciousness in the Other. It would suffice to replace Turing’s intelligence with Hegel’s self-consciousness to reveal the similarity between these two approaches to the Theory of the Mind. In Hegel’s account, however, the framing power structures remain obvious, manifest, and unavoidable (an aspect that pleased Marx), while in the Turing Test politics evacuates the field (an aspect that pleases the artificial intelligence community today).
The Imitation Game was sketched for the first time by Turing in his 1948 paper “Intelligent Machinery,” wherein, more compellingly, he proposed the idea of an unorganized machine that would be able to learn by continuous interferences in its open structure.9 Turing made a direct parallel between the learning machine and the cortex of an infant, in which he cheerfully sided with the fallibility of machines: “It would be quite unfair to expect a machine straight from the factory to compete on equal terms with a university graduate.”10 In a 1947 lecture he went so far as to equate intelligence with fallibility: “If a machine is expected to be infallible, it cannot also be intelligent.” Turing’s hypothesis, which puzzled many mathematicians, was that a machine could be programmed to make mistakes like humans do: a statement that would reduce Gödel’s incompleteness theorem to irrelevance.11 Turing had different conceptions of machine intelligence during his life, but it is the Turing Test that has monopolized the attention of the philosophers of mind and galvanized the neovitalism of artificial intelligence.
Once Turing developed the hypothesis that a machine could imitate human mistakes, his argument risked deteriorating in a toxic loop, since it envisions machine intelligence as cosmic plagiarism mirroring the routines of an already bored-to-death humanity. In this respect, the Turing Test is a premonition of universal industrial imitation, a Universal Machine that would replace not only the previous division of manual and mental labor but would be able to replicate all imaginable divisions of labor. The Turing Machine would be better defined as a Social Imitation Machine, as its power is revealed in the power to imitate, amplify, and accumulate social relations. In the belly of data centers, machine intelligence is already emerging as a novel perspective on suprahuman and invisible clusters of social data, not as the quality of imitating human features and feelings. Machine intelligence is not anthropomorphic, but sociomorphic: it imitates and feeds on the condividual structures of society rather than the individual ones.
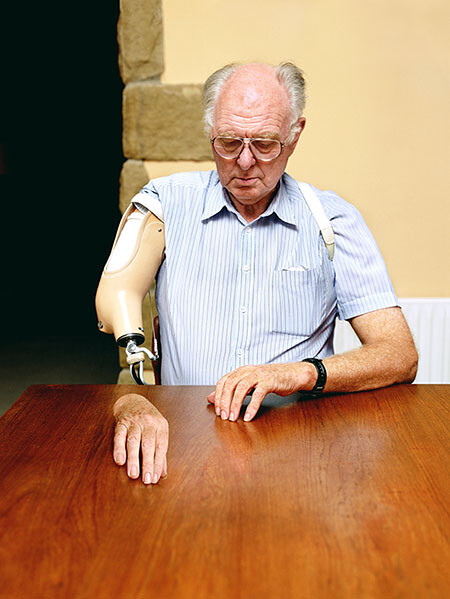
The Metastable Mind and Its Technological Individuation
After WWII the French philosopher Gilbert Simondon attempted to develop a philosophy of the mind that would depart both from the organism-form inherited from German vitalism (still influential at the time) and the information-form that had just been introduced by North American cybernetics.12 These two polarities had long been operative in French philosophy, at least since Descartes and the dispute over the machine body. Against the primacy of the new technical form and the old Lebensform, Simondon envisioned a “metastable mind” constructed in a triangular space between the biological, the technological, and the social, giving the latter a leading, even constituent, role. Simondon was not concerned with individualized structures (brain, organism, technology, society) so much as with the collective process of individuation (the old principium individuationis) that made these hegemonic structures possible. What makes a mind? Simondon considered both mechanicism (later: informationalism) and holism (later: organicism and Gestalttheorie) as enclosures of a process that must be kept conceptually open. In Simondon the actual mind emerges to “solve the problems posed to the living being”13 by the surrounding world and it always reinvents itself in a process open towards the social.
In Simondon the construction of the mind (or psychic individuation) is not originary but a process of collective individuation: the mind is constructed with signs, objects, and artifacts of the external and social world. In this sense, we have all developed a “technical mentality” (yet developing a technical mentality, nota bene, does not mean employing technology as a model of the mind). The Marxist philosopher Paolo Virno has underlined the similarity between Simondon’s notion of individuation and Soviet psychologist Lev Vygotsky’s work on the development of language skills and thought in the child.14 For Vygotsky, the faculty of inner speech in the child (and so the Theory of Mind, we may add) is produced by the power of the individuation of social language, and this process continues in adult life.15 Similarly for Simondon, individuals are never completely individuated but maintain an excess of “transindividuality” that distinguishes them from technological artifacts as much as from animals.
In terms of logic forms, Simondon struggled to find a concept that could synthesize and overcome the notions of both organic Gestalt (inherited via German Naturphilosophie) and technological information (received from cybernetics). Simondon called “transduction” this concept that could cross the “transindividual” psyche without enclosing it in either an organic or a technological form, leaving its excessive potentiality open. Transduction is not the idea of the multiple realizability of the One Mind, but the idea of multiple genealogies of mentalization that keep on innervating the fabric of the world, along the lines of that parallel ontology that Deleuze would term “transcendental empiricism.” One model of the mind can be transducted into another, but the process is not frictionless and free of conflict like in the idea of a Turing-complete universe. The historical translation of a model of the mind into another is not only a technological problem but still a political one.
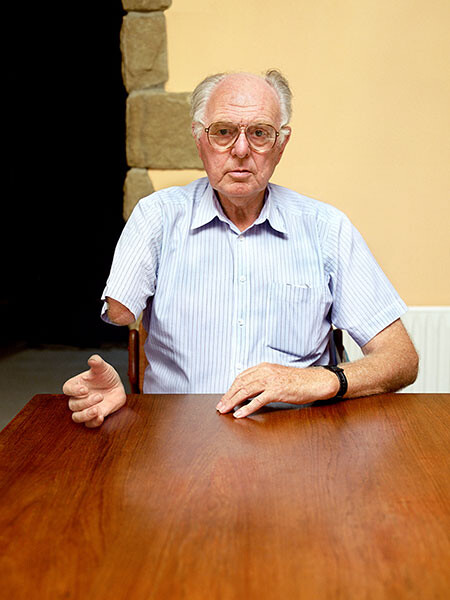
Simondon’s work has also inspired discussions in the Artificial (General) Intelligence community. The problem of psychic individuation in the debate on machine intelligence and the Turing Test can be translated as the problem of mentalization or “encephalization,” to borrow a term from evolutionary biology. How do you recognize a mind? Some answer by saying that you recognize a mind if you know how to construct it. Instead of starting with the question “what does it mean to be intelligent?” David Weinbaum and Viktoras Veitas from the Global Brain Institute in Brussels ask “what does it mean to become intelligent?” Drawing from Simondon’s idea of individuation, they have come up with the paradigm of “open-ended intelligence,” which reiterates the old idea of intelligence as an emergent property of natural systems.
Open-ended intelligence is a process where a distributed population of interacting heterogeneous agents achieves progressively higher levels of coordination. By “coordination” here we mean the local resolution of disparities by means of reciprocal determination, which brings forth new individuals in the form of integrated groups of agents (assemblages) that exchange meaningful information and spontaneously differentiate (dynamically and structurally) from their surrounding milieu. This kind of intelligence is truly general in the sense that it is not directed or limited by a given, a priori goal or challenge. Moreover, it is intrinsically and indefinitely scalable, at least from a theoretical point of view. We see open-ended intelligence manifesting all around us and at many scales: primarily in the evolution of life, in the phylogenetic and ontogenetic organization of brains, in lifelong cognitive development and sense-making, and in the self-organization of complex systems from slime molds, fungi, and beehives to human sociotechnological entities.16
This description of open-ended intelligence appears to conflate the two forms that Simondon actually attempted to overcome: the biological and the technological. In other words, it seems like a naturalization of machine intelligence—the equating of machine intelligence with the living. In explaining the biomorphic fallacy, it is helpful to remember that we did not design the airplane to fly as birds do, and so machine intelligence need not follow the path of some biological ancestor. Even so Weinbaum and Veitas stress that “many believe that one day in the foreseeable future the internet will awaken and become a conscious aware super-intelligent entity. Some even claim that this is already happening.”17 Such professions of computational animism seem like an a priori condition for being accepted in these tech communities. Animism keeps on haunting artificial intelligence: non-biomorphic notions of machine intelligence are urgently needed.
More importantly, the idea of pancomputationalism in nature mystifies a basic reality: computation is actually an economic process, one that aims at extracting valuable information and discarding useless information. In this sense computation is also a process of capitalization. And so to assert, as Stephen Wolfram and Ray Kurzweil do, among others, that all atoms encode and compute, is to equate capital and nature.18 Atoms do not get rid of useless information in order to escalate to a higher degree of complexity. Apologies to Kurzweil’s vision of a computational sublime standing before a stormy ocean, as reported in the documentary Transcendent Man (2009), but the molecules of water in the ocean do not compute.19 That’s just us.
The Artificious Intelligence of the Market
Markets have been a place of vernacular artificial intelligence for a long time. The Austrian economist Friedrich Hayek believed that the market is the ground of a preconscious and transindividual knowledge that needs neither state centralization (like in socialist planning) nor formulation in objective economic laws (Hayek is the godfather of the notorious Chicago School but he maintained, interestingly, a great interest in psychology and neuroscience throughout his life). The infrarationality of the market is for Hayek far beyond the comprehension of the individual as much as the state: “The economic problem is … a problem of the utilization of knowledge not given to anyone in its totality,” he wrote in his 1945 seminal text “The Use of Knowledge in Society.”20 Hayek castigated the ambitions of statistics and, in this way, implicitly, also those of computation: “[The] sort of knowledge with which I have been concerned is knowledge of the kind which by its nature cannot enter into statistics and therefore cannot be conveyed to any central authority in statistical form.”21 Hayek believed that prices are the best signals for condensing and transmiting all necessary economic information: they work, in fact, like a collective computer (“system of telecommunications” in the wording of 1945):
It is more than a metaphor to describe the price system as a kind of machinery for registering change, or a system of telecommunications which enables individual producers to watch merely the movement of a few pointers, as an engineer might watch the hands of a few dials, in order to adjust their activities to changes of which they may never know more than is reflected in the price movement.22
Hayek might have been the first to introduce a modern (i.e., functional) notion of information: it must be remembered that Claude Shannon defined the mathematical measure of information only in 1948, the same year in which Norbert Wiener published his book Cybernetics.23 Nevertheless, Hayek described the market as a cognitive apparatus, in a strong similarity with early cybernetics and long before theories of the knowledge society and cognitive capitalism. Since Adam Smith, the topos of the “invisible hand” has been repeated to describe the virtues of the free market, but the expression “invisible mind” would be more accurate for framing such a distributed and spontaneous coordination of prices. In Hayek’s vision the market seems to be run by an invisible general intellect that cannot be objectified in any machinery, but only in commodity prices. Yet such idealism has been contradicted, most recently, by what has been called algorithmic capitalism. Today, companies like Uber and Airbnb are able to centralize price calculation through their global databases in real time. In this respect algorithmic capitalism is the rise of a third paradigm: the worst nightmares of both centralized planning and free-market deregulation, which come true under the rule of one master algorithm designed by the mathematicians and engineers of machine learning.24
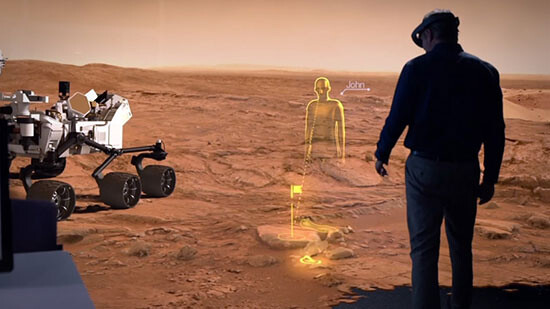
Capital as Encephalization
Even if artificial intelligence never awakes one day as a sentient being, there are already millions of machine learning algorithms that day-to-day scavenge gigantic data centers of social data to detect correlations, extract patterns, distil norms, predict tendencies, and make metadata mug shots of the population as a whole. Machine intelligence is not biomorphic—it will never be autonomous from humankind and, for sure, from the difficulties of capital, since it is a functional component of industrial planning, marketing strategies, securitarian apparatuses, and finance.
Machine intelligence is sociomorphic, but not in a good way. Machine intelligence mirrors social intelligence in order to control the latter. The Turing universe is one of those magnifying mirrors, and it makes the collective body look grotesque, disproportioned, abnormalized by the glitches of computational power. We feed algorithms our racist, sexist, and classist biases and in turn they warp them further. As Marx knew, absent political action, machines do not just replace but amplify previous divisions of labor and social relations. Turing machines are no exception: datasets of populations educated in fundamentalism project an even more fundamentalist machine intelligence pattern. Machine intelligence is then anamorphic: at the 2016 conference “Tyranny of the Algorithms? Predictive Analytics and Human Rights,” even the New York Police Department acknowledged the class and racial bias produced by its crime-predicting algorithms.25 The Microsoft Twitter bot that turned its posts into fascist rants is another example of how machine intelligence can be easily misdesigned, especially if it is designed by companies with dubious standards.
Machine intelligence should become sociomorphic in a good way. Machine learning and data analytics do manage to unveil a superior social dimension that is intrinsic to any piece of digital information and that has been intangible and inaccessible until now. The techniques of data visualization and navigation finally give an empirical form to the collective mind and to modern concepts of collective agency, such as Marx’s general intellect, Foucault’s episteme, and Simondon’s transindividual, which have been so far pretty abstract and invisible to the eye of the individual mind. Alternative and progressive uses of machine intelligence are always easy to imagine but difficult to realize. Alternative techniques of data mining are being explored today by an emerging field known as “data activism,” which fights for social justice, human rights, and equal access to education and welfare. In this respect, the AGI community astonishingly lacks the kind of basic ABCs of politics that can be found in other tech communities (see the history of the Free Software Foundation and Electronic Frontier Foundation). Here’s a short manifesto of transcendental empiricism for the AGI community: not trying to realize the One Mind, like who like to replace Chairman Mao with the role played by Artificial Intelligence, but trying to construct a metastable collective intelligence that would be politically more ‘intelligent’ than the ideal of the One Mind.
Within the regime of cognitive capitalism, computation is coming to occupy a growing hegemonic role: machine intelligence is replacing a complex division of mental and manual labor and encephalizing collective intelligence and social behavior into data centers. The reductionist philosophy of mind promoted by the artificial intelligence community is organic, therefore, to the issue of capital qua computation and cognition. Historically, the rising hegemony of the paradigm of machine learning marks the final transition from cognitive capitalism to computational capitalism and centralized forms of machine intelligence. As much as the British industrial class worshipped the steam engine as the idol of a new society and the concretion of power, likewise the new vectoralist class of artificial intelligence is starting to animate supercomputation.26 In this respect, the movie Esiod 2015 by German artist Clemens von Wedemeyer is right in imagining that if the Singularity ever happens, it will be a financial one: it will most likely be your family bank that becomes an autonomous sentient entity.27 To still believe in the myth of the autonomy of artificial intelligence is to support the autonomy of capital against the autonomy of society.
Capitalism is a process of encephalization, that is, a process of the accumulation of human intelligence. This process had already begun in the industrial factory, when Charles Babbage designed the Analytical Engine with the idea absorbing and automating the division of mental labor. Simondon was the first to recognize that the industrial machine was already an infomechanical relay separating the source of energy from information—that is, from the intelligence of the worker. As British historian of science Simon Schaffer recounts:
The word “intelligence” refers both to signals received from without and to the capacity to register and interpret these signals. In early nineteenth-century Britain the word intelligence simultaneously embodied the growing system of social surveillance and the emerging mechanisation of natural philosophies of mind … To make machines look intelligent it was necessary that the sources of their power, the labor force which surrounded and ran them, be rendered invisible … The replacement of individual human intelligence by machine intelligence was as apparent in the workshop as in the engines. This task was both politically and economically necessary.28
Computation could have had a different destiny, but it quickly slipped under the dominion of capital, reinforcing a new stage of power. Computation secularized the human mind, only to industrialize and venerate, immediately afterward, the automation of mental labor as artificial intelligence (according to the classic oscillation of desubjectification and resubjectifation). Supercomputation displaced the subject of Western humanism even further from the center of thought, but only so that capital might think in its place. As the root of the word suggests (caput in Latin means “head”), capital is a vast process of encephalization: it continuously returns to destroy and reconstruct again its own head.
In this text the term “artificial intelligence” refers to anthropomorphic and biomorphic models of intelligence, whereas the term “machine intelligence” refers to a form of intelligence that does not resemble features of the living (including human feelings and “consciousness”). A more secular definition of machine intelligence will help, hopefully, to disclose posthuman and antinormative correlations in social data rather than to reinforce the individual and social norms of class, gender, and race.
Theodor Adorno and Max Horkheimer, Dialectic of Enlightenment (Stanford: Stanford University Press, 2002), 21. Quoted in Anselm Franke, Animism (Berlin: Sternberg Press, 2010).
Stephen Wolfram, A New Kind of Science (Champaign, Il: Wolfram Media, 2002), 845.
Musk’s concerns about AI probably originate, by the way, from the unfair practices of Google, his largest corporate competitor. See Rich McCormick, “Elon Musk: There’s only one AI company that worries me,” The Verge, June 2, 2016 →.
See Andrew Pickering, The Cybernetic Brain: Sketches of Another Future (Chicago: University of Chicago Press, 2010), chap. 1.
The German biologist Jakob von Uexküll described the relation between an animal’s nervous system (Innenwelt) and the outside world (Außenwelt) as a “functional circle” (Funktionskreis). Similar to the Funktionskreis, the feedback loop of cybernetic systems is conceived as a circulation of information and response to an external stimulus.
David Bates, “Unity, Plasticity, Catastrophe: Order and Pathology in the Cybernetic Era,” in Catastrophe: History and Theory of an Operative Concept, eds. Andreas Killen and Nitzan Lebovic (Berlin: De Gruyter, 2014).
Norbert Wiener, Cybernetics: or Control and Communication in the Animal and the Machine (Cambridge, MA: MIT Press, 1948), 30.
The model was inspired by McCulloch and Pitt’s model of neural networks: their work was not referred to, but Turing’s paper was not published either. See Warren McCulloch and Walter Pitts, “A logical calculus of the ideas immanent in nervous activity,” Bulletin of Mathematical Biophysics, vol. 5, no. 4 (1943).
Alan Turing, “Intelligent Machinery” (1948), in The Essential Turing, ed. Jack Copeland (Oxford: Oxford University Press, 2004), p. 421.
Alan Turing, “Lecture on the Automatic Computing Engine,” (1947), in ibid., 394. See Andrew Hodges, “Alan Turing,” Stanford Encyclopedia of Philosophy, ed. Edward Zalta, Winter 2013 edition: “Once the possibility of mistakes is admitted, Gödel’s theorem becomes irrelevant. Mathematicians and computers alike apply computable processes to the problem of judging the correctness of assertions; both will therefore sometimes err, since seeing the truth is known not to be a computable operation, but there is no reason why the computer need do worse than the mathematician.”
Simondon had a profound influence on Gilles Deleuze, who dedicated to him one of his rare book reviews. See Gilles Deleuze, “Gilbert Simondon: L’Individu et sa genèse physicobiologique,” Revue philosophique de la France et de l’étranger, CLVI:1–3 (1966).
Gilbert Simondon, “Individuation in the Light of the Notions of Form and Information.” Quoted in Andrea Bardin, Epistemology and Political Philosophy in Gilbert Simondon (Dordrecht: Springer, 2015), 70.
Paolo Virno, When the Word Becomes Flesh: Language and Human Nature (Los Angeles: Semiotexte, 2015). See also Charles Wolfe, “De-ontologizing the Brain: from the fictional self to the social brain,” CTHEORY, 2007 →.
Lev Vygotsky, Thought and Language (Cambridge, MA: MIT Press, 1986 (1934)).
David Weinbaum and Viktoras Veitas, “Open-Ended Intelligence: The Individuation of Intelligent Agents,” Journal of Experimental & Theoretical Artificial Intelligence, 2016: 1–26.
Ibid.
Pancomputationalism is also addressed by French philosopher Michel Serres. See Matteo Pasquinelli, “On Solar Databases and the Exogenesis of Light,” in “Supercommunity,” special issue, e-flux journal 65 (May 2015) →.
Thanks to Lorenzo Sandoval for pointing to this section of the documentary.
Friedrich Hayek, “The Use of Knowledge in Society,” American Economic Review, 1945: 520.
Ibid., 524.
Ibid., 527.
Claude Shannon, “A Mathematical Theory of Communication,” Bell System Technical Journal, vol. 27, no. 3, 1948.
“Master algorithm” is an expression used in machine learning. See Pedro Domingos, The Master Algorithm (New York: Basic Books, 2015).
The conference took place at New York University, March 21–22, 2016.
For an account of the British industrialist class’s cult of the steam engine, see Andreas Malm, Fossil Capital: The Rise of Steam Power and the Roots of Global Warming (London: Verso Books, 2016).
Esiod 2015, directed by Clemens von Wedemeyer, 39 min, Austria/Germany, 2016.
Simon Schaffer, “Babbage’s Intelligence: Calculating Engines and the Factory System,” Critical Inquiry, vol. 21, no. 1 (1994).