Post-truth politics is the art of relying on affective predispositions or reactions already known or expressed to stage old beliefs as though they were new. Algorithms are said to capitalize on these predispositions or reactions recorded as random data traces left when we choose this or that music track, this or that pair of shorts, this or that movie streaming website. In other words, the post-truth computation machine does not follow its own internal, binary logic of either/or, but follows instead whatever logic we leave enclosed within our random selections. To the extent that post-truth politics has a computational machine, then, this machine is no longer digital, because it is no longer concerned with verifying and explaining problems. The logic of this machine has instead gone meta-digital because it is no longer concerned with the correlation between truths or ideas on the one hand, and proofs or facts on the other, but is instead overcome by a new level of automated communication enabled by the algorithmic quantification of affects.
The meta-digital machine of post-truth politics belongs to an automated regime of communication designed to endlessly explore isolated and iterated behaviors we might call conducts. These are agencies or action patterns that are discrete or consistent enough to be recognized by machine intelligence. Post-truth political machinery employs a heuristic testing of responses interested in recording how conducts evolve, change, adapt, and revolt. This is not simply a statistical calculation of probabilities following this or that trend in data usage, but involves an utter indifference towards the data retrieved and transmitted insofar as these only serve as a background. And yet the content of the data is not trivial. On the contrary, the computational machine entails a granular analysis of data on behalf of algorithms, which rather open up the potential of content to be redirected for purposes that are not preknown. In other words, this computational indifference to binary problem-solving coincides with a new imperative: technological decisionism, which values making a clear decision quickly more than it does making the correct one. For decisionism, what is most decisive is what is most correct. When Mussolini gives a speech in parliament, in 1925, taking full responsibility for the murderous chaos his regime has created, and challenging his opponents to remove him anyway, he is practicing decisionism at the expense of binary logic, which would dictate that if Mussolini is responsible, then he should resign. Instead, the dictator declares that he is responsible and that he will stay. Today it is our machines who make these speeches for us.
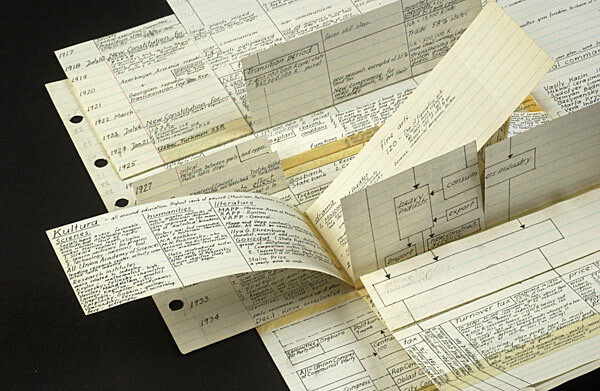
George Maciunas, Learning Machine, 1969. Paper.
Decide Not to Decide and Results Will Come
The history of communication that obtains results by undoing truths and by fabricating rather than discovering facts must include at least three historical moments in the development of machine intelligence and the formation of the meta-digital machine. First, the period from the 1940s to the 1960s, involving the rise of the cybernetic infrastructure of communication and the introduction of computational logic into decision-making procedures; second, the 1970s and 1980s, which saw a shift towards interactive algorithms and expert and knowledge systems; and third, from the post-80s to the post-2000s, which were characterized by a focus on intelligent agents, machine-learning algorithms, and big-data logic. As these forms of automated intelligence have entered the social culture of communication, they have also become central to a critical theory of technology that has incessantly warned us against the automation of decision, where information processing, computational logic, and cybernetic feedbacks replace the very structure, language, and capacity of thinking beyond what is already known.
In the essay “The End of Philosophy and the Task of Thinking” (1969), Martin Heidegger argues that since the late 1940s, the advance of cybernetics—a technoscience of communication and control—has demarcated the point at which Western metaphysics itself reaches a completion.1 This means not only that philosophy becomes verifiable and provable through testing, but also that scientific truths become subsumed to the effectiveness of results. By replacing judgment based on the supposition of categories with the efficiency of truth-states carried out by machines, the instrumental reasoning of cybernetics fully absorbs Western metaphysics. No longer could technoscience remain at the service of philosophy. Here, ideas are not simply demonstrated or proved, but processed as information. The new technoscience of communication activates a new language of thought embedded in the information circuits of input and output, according to which actions are programmed to achieve a series of results.
If, according to Heidegger, the end of philosophy is “the gathering in of the most extreme consequences,” it is because the development of the sciences and their separation from philosophy has led to the transformation of philosophy into “the empirical science of man.”2 Nowhere is this more tangible than with the advance of cybernetics and its concerns with “the determination of man as an acting social being. For it is the theory of the steering of the possible planning and arrangement of human labor. Cybernetics transforms language into an exchange of news. The arts become regulated-regulating instruments of information.”3 As philosophy becomes a science that intercommunicates with others, it loses its metaphysical totality. The role of explaining the world and the place of “man in the world” is finally broken apart by technology.
Under this new condition of the techno-erasure of metaphysical truth, Heidegger insists that the new task of thinking shall lie outside the distinction of the rational and the irrational. Since thinking always remains concealed within the irrationality of systems, it cannot actually be proven to exist. From this standpoint, and echoing Aristotle, he poses the question of how it is possible to recognize whether and when thinking needs a proof, and how what needs no proof shall be experienced.4 For Heidegger, however, only un-concealment—the condition in which thinking cannot be unconcealed—would coincide with the condition of truth. Here, truth will not imply the certainty of absolute knowledge—and therefore it will not belong to the realm of scientific epistemology. From this standpoint, since the cybernetic regime of technoscientific knowledge mainly concerns the achievement of results, it can tell us nothing about truth, as the latter entails the un-concealment of what cannot be demonstrated—because thinking always hides in the irrationality of systems. Truths, therefore, must remain outside what is already known. This is why, in the age of meaningless communication, according to Heidegger, one must turn the task of thinking into a mode of education in how to think.5
It is precisely a new envisioning of how to think in the age of automated cognition that returns to haunt post-truth politics today. We are at an impasse: unable to return to the deductive model of ideal truths, but equally unable to rely on the inductive method or simple fact-checking to verify truth. How do we overcome this impasse? It is difficult to shift perspective on what technopolitics can be without first attempting to disentangle this fundamental knot involving philosophy and technoscience, which is still haunted by Heidegger’s proposition that the transformation of metaphysics—of the un-demonstrable condition of thinking—into cybernetic circuits of communication demands an articulation of thinking outside reason and its instrumentality.
As such, the inheritance of this critique of thought still seems to foreclose the question of instrumental reasoning today, whereby artificial-intelligence machines, or bots, have recoded critical perspectives about the ideology of truth and the fact-checking empiricism of data. Instead of declaring the end of metaphysical thinking and its completion in instrumentality, it seems important to reenter the critique of instrumental reasoning through the backdoor, reopening the question of how to think in terms of the means through which error, indeterminacy, randomness, and unknowns in general have become part of technoscientific knowledge and the reasoning of machines.
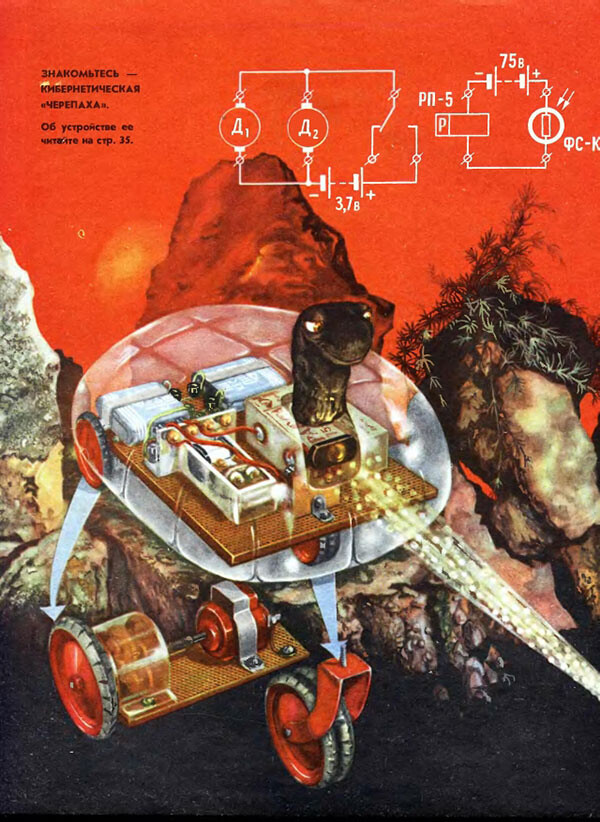
Cover of a Soviet manual documenting the construction of the “cybernetic tortoise,” 1969.
Learning to Think
To do so, one could start looking more closely at the historical attempts in cybernetics and computation between the late 1940s and the 1980s to bring forward models of automated intelligence that did not rely on the deductive logic of known truths. As the application of inductive data-retrieval and heuristic testing shifted the focus of artificial intelligence research from being a mode of validation to becoming a mode of discovery, critical theory’s assumptions that technoscience mainly exhausted metaphysics with the already thought—or with mere communication—had to be revised. It is precisely the realization of the ontic limit of technoscience that pushed cybernetics and computation away from symbolic rational systems and towards experimenting with knowing how—that is, with learning how to learn—which is now central to the bot-to-bot curatorial image of social communication in the age of post-truth and post-fact. With cybernetics and computation, the rational system of Western metaphysics is not simply realized or actualized, but becomes rather wholly mutated. Here, the eternal ground of truth has finally entered the vicissitudes of material contingencies. Cybernetic instrumentality replaces truth as knowledge with the means of knowing, and announces a metaphysical dimension of machine knowledge originating from within its automated functions of learning and prediction. And yet, in this seamless rational system, one can no longer conceive of thinking as what is beyond or what remains unconcealed in the invisible gaps of a transparent apparatus of communication. Instead, it seems crucial to account for the processing of the incomputable (of indeterminate thinking) in machine reasoning as a modern condition by which instrumentality also announced that means are a step beyond what they can do. This transcendental instrumentality opens the question of the relation between doing and thinking which is at the core of the critique of technologies. Hence, while the contemporary proliferation of post-truth and post-fact politics appears to be a consequence of the end of Western metaphysics as initiated by cybernetics and computation, the consequences of a metaphysic of machines is yet to be fully addressed in terms of the origination of a nonhuman thinking. But what does it mean that machines can think? Hasn’t the critique of technology, from Heidegger to Deleuze and even Laruelle,6 indeed argued that the immanence of thought passes through nonreflective and non-decisional machines? And if so, is post-truth, post-fact politics indeed just the most apparent consequence of how irrational thinking pervades the most rational of systems? And yet, there is the possibility of addressing the question of inhuman thinking otherwise from within the logic of machines and in terms of the origination of a machine epistemology whereby complex levels of mediation, and not an immediacy between doing and thinking, is at stake.
Already in the 1940s, Walter Pitts and Warren McCulloch’s influential paper on neural nets proposed to replace the deductive model of mathematical reasoning based on symbolic truths with heuristic methods of trial and error that would allow machines to learn at an abstract level and not simply through sensorimotor feedback response. As historian of cybernetics Ronald Kline points out, these mathematical neural nets already focused on the levels of randomness in a network rather than simply relying on the homeostatic function of feedback.7 However, the Pitts and McCulloch neural model of knowledge mainly coincided with a connectionist view of intelligence, associating artificial neurons with mathematical notations, bonding the biological firing of neurons to the emanation of concepts. This model used the learning behavior of algorithms as a representational tool to mainly clarify cognitive functions.8 However, since a single neuron could only compute a small number of logical predicates, and was thus insufficient for explaining the complexity of parallel thinking, this model hit a dead end with post-1980s research in AI.
It was only between the late 1980s and 1990s, after the so-called “AI winter,” that new efforts at automating reasoning employed sub-symbolic views of intelligence that largely adopted nondeductive and heuristic methods of testing results that allowed algorithms to learn from uncertain or incomplete information. Through inductive methods of data retrieval and transmission, algorithms would learn—or train in time—from a relatively small background of data. Instead of simply validating results according to given axioms, algorithms had instead become performative of data; that is, through recursive and probabilistic calculations, algorithms would not only search for information, but also extract and combine patterns.
By the late ’90s, the rule-bound behavior of algorithms had become evolutionary: they adapted and mutated over time as they retrieved and transmitted data. Experiments in genetic and evolutionary algorithms eventually transformed machine learning as a means of validating proof, creating neural networks equipped with hidden layers corresponding to the state of a computer’s memory that run another set of instructions in parallel.9 In general, neural networks with a greater depth of layers can execute more instructions in sequence. For instance, the mode of machine learning called “back-propagation” train networks with hidden layers, so that simpler computational units can evolve. We know that back-propagation algorithms are a data-mining tool used for classification, clustering, and prediction. When used in image recognition, for instance, this mode of machine learning may involve training the first layer of an algorithm to learn to see lines and corners. The second layer will learn to see the combination of these lines to make up features such as eyes and a nose. A third layer may then combine the lines and learn to recognize a face. By using back-propagation algorithms, however, only the features that are preselected will be expressed. As a consequence, the backdrop of data to be mined is already known, and as such gender and race biases, for instance, are either already encoded in the data sets or are blind, that is invisible to the algorirthms. Here, far from granting an objective representation of data, machine learning has rather been seen as an amplifier of existing biases, as revealed by the association of words and images in automated classification and prediction.10
Instead of a top-down programming of functions, adaptive algorithms in neural networks process data at increasingly faster speeds because they retrieve and transmit data without performing deductive logical inferences. However, according to Katherine Hayles, algorithmic intelligence, more than being mindless, should rather be understood as a nonconscious form of cognition, solving complex problems without using formal languages or deductive inference. By using low levels of neural organization and iterative and recursive patterns of preservation, these algorithms are inductive learners; that is, they develop complex behavior by retrieving information from particular data aggregates. However, Hayles points out that emergence, complexity, adaptation, and the phenomenal experience of cognition do not simply coincide with the material processes or functions of these elements of cognition.11 Even if algorithms perform nonconscious intelligence, it does not mean that they act mindlessly. Their networked and evolutionary learning cannot simply be understood in terms of their material functions, or to put it another way, according to their executive functions.
In contrast to Lorraine Daston’s vision that algorithmic procedures are mindless sets of instructions that replaced logos with ratio,12 Hayles’s argument about nonconscious cognition suggests that algorithmic procedures are transformed in the interaction between data and algorithms, data and metadata, and algorithms and other algorithms that define machine learning as a time-based medium in which information vectors converge and diverge constantly. Since, according to Hayles, machine learning is already a manifestation of low-level activities of nonconscious cognition performed at imperceptible or affective speeds, it is not possible to argue that cognition is temporally coherent, linking the past to the present or causes to effects. According to Hayles, information cannot simply be edited to match expectations. Instead, the nonconscious cognition of intelligent machines exposes temporal lapses that are not immediately accessible to conscious human cognition. This is an emergentist view of nonconscious cognition that challenges the centrality of human sapience in favor of a coevolutionary cognitive infrastructure, where algorithms do not passively adapt to data retrieved but instead establish new patterns of meaning by aggregating, matching, and selecting data. From this standpoint, if the inductive model of trial and error allows computational machines to make faster connections, it also implies that algorithms learn to recognize patterns and thus repeat them without having to pass through the entire chain of cause and effect and without having to know their content.
However, as algorithms have started training on increasingly larger data sets, their capacity to search no longer remains limited to already known probabilities. Algorithms instead have become increasingly instrumental of data, experimenting with modes of interpretation that Hayles calls “techno-genesis,” pointing towards an instrumental transformation of “how we may think.”13
In the last ten years, however, this instrumental transformation has also concerned how algorithms may think amongst themselves. Since 2006, with deep learning algorithms, a new focus on how to compute unknown data has become central to the infrastructural evolution of artificial neural networks. Instead of measuring the speed of data and assigning it meaning according to how frequently data are transmitted, deep-learning algorithms rather retrieve the properties of a song, an image, or a voice to predict the content, the meaning, and the context-specific activities of data. Here, algorithms do not just learn from data, but also from other algorithms, establishing a sort of meta-learning from the hidden layers of the network, shortening the distance from nodal points while carrying out a granular analysis of data content. From this standpoint, machine-learning algorithms do not simply perform nonconscious patterns of cognition about data, exposing the gaps in totalizing rational systems, but rather seem to establish new chains of reasoning that draw from the minute variations of data content to establish a machine-determined meaning of their use.
This focus on content-specific data is radically different from the conception of information in communication systems in the 1940s and the postwar period. For Claude Shannon, for instance, the content of data was to be reduced to its enumerative function, and information had to be devoid of context, meaning, or particularities. With deep learning, big data, and data mining, algorithms instead measure the smallest variations in content- and context-specific data as they are folded into the use of digital devices (from satellites to CCTV camera, from mobile phone to the use of apps and browsing). Indeed, what makes machine learning a new form of reasoning is not only the faster and larger aggregation of data, but also a new modality of quantification, or a kind of qualitative quantification based on evolving variations of data. This is already a transcendental quality of the computational instrument, exposing a gap between what machines do and how they think.
In other words, deep-learning algorithms do not just learn from use but learn to learn about content- and context-specific data (extracting content usage across class, gender, race, geographical location, emotional responses, social activities, sexual preferences, music trends, etc.). This already demarcates the tendency of machines to not just represent this or that known content, or distinguish this result from another. Instead, machine learning engenders its own form of knowing: namely, reasoning through and with uncertainty. The consequence of this learning, it can be argued, seems to imply more than the unmediated expression of an immanent thought, or the reassuring explosion of the irrational within rational systems. Machine learning rather involves augmented levels of mediation, where uncertainty is manifested in terms of the incomputable forms of algorithmic automation, as that which does not simply break the calculation, the quantification, the numerical ordering of infinities. Instead, incomputables enter the complex sizing of mediation, involving the structuring of randomness on behalf of the algorithmic patterning of indeterminacies. This implies that machine learning should not be solely considered in terms of what algorithms do as a biased model of the reproduction of data-usage, data-context, and data-meaning. At the same time, however, one should resist the temptation to consider algorithms as mere placeholders that allow the manifestation of the nonconscious or irrational potentialities of thinking. Instead, I want to suggest that the very general principle of learning in machines should be critically addressed in terms of a nascent transcendental instrumentality: what machines do does not and should not coincide with the possibilities of machine thinking. This implies not only that thinking transcends mere pragmatics, but also, and more importantly, that a pragmatic reasoning aspire to build thought by conceding that future modes of actions can transform the conditions of knowing how. Here, the irrational is not outside reasoning but discloses the alien possibilities that a general practice of reasoning offers in terms of a mediation of technosocial changes and actions.
If this aspirational critique of machine learning asks for a change in perspective on the possibilities for a critical theory of automated thinking, one cannot however overlook the fact that algorithmic control and governance do involve the micro-targeting of populations through the construction of alternative facts aimed at reinforcing existing beliefs. At the same time, the evolutionary dynamics of learning machines show that the time of computation, including the hidden layers of a growing network, also forces algorithms to structure randomness beyond what is already known. For instance, if a machine is fed with data that belongs to already-known categories, classes, and forms, when the computational process starts, these data become included in the algorithmic search for associations that bring together smaller parts of data, adding hidden levels of temporalities to the overall calculation. This results in the algorithmic possibilities of learning beyond what is inputted in the system.
From this standpoint, if we maintain that algorithms are mindless and nonconscious, we are also arguing that computational control only results in the reproduction of the ideological and discursive structure of power that data are said to uphold. In other words, whether it is suggested that the machine architecture of algorithms and data is another form of ideological design (imbued with human decisions) or that machines are ultimately mindless and can thus act empirically (simply as data checkers), what seems to be missing here is a speculative critique of machine learning that envisions machines as something more than mere instances of instrumental reasoning—vessels of knowledge that can at best perform Western metaphysical binaries, deductive truths, and inductive fact-checking at a faster pace. While this view reveals that the nexus of power and knowledge is now predicated on existing beliefs and granular aggregations of data, it does not offer a critical approach to technology that can sweep away the Heideggerian prognosis that technoscience has transformed the task of thinking, replacing truth with the effectiveness of rule-bound behavior.
A critical theory of automation should instead start with an effort to overturn the autopoietic dyad of instrumental reasoning, where machines either execute a priori reasoning or reduce the rule of reason (law and truth) to brute force and reactive responses. In other words, this critique should reject the view that technoscience completes Western philosophy’s dream of reason and rather account for the overturning of technoscientific knowledge and of philosophical reason activated with and through experimentations with the limits of artificial intelligence. Here, the microtargeting of populations involves not only the reproduction of biases in and through data aggregates, but the algorithmic elaboration of any possible data to become racialized, gendered, and classified as a potential enemy under certain circumstances.
But how do we address these correlations between the end of truth and fact and the transformation of cybernetic binary states into forms of nonconscious cognition and meta-digital learning processing, where algorithms do not just perform data content, but learn how to learn and thus how to include indeterminacy in reasoning? Is it enough to blame the mindless technoscientific quantification of biased beliefs and desires, or is it possible to engage in a materialist theorization of technicity, starting from a close engagement with the means by which thinking thinks?
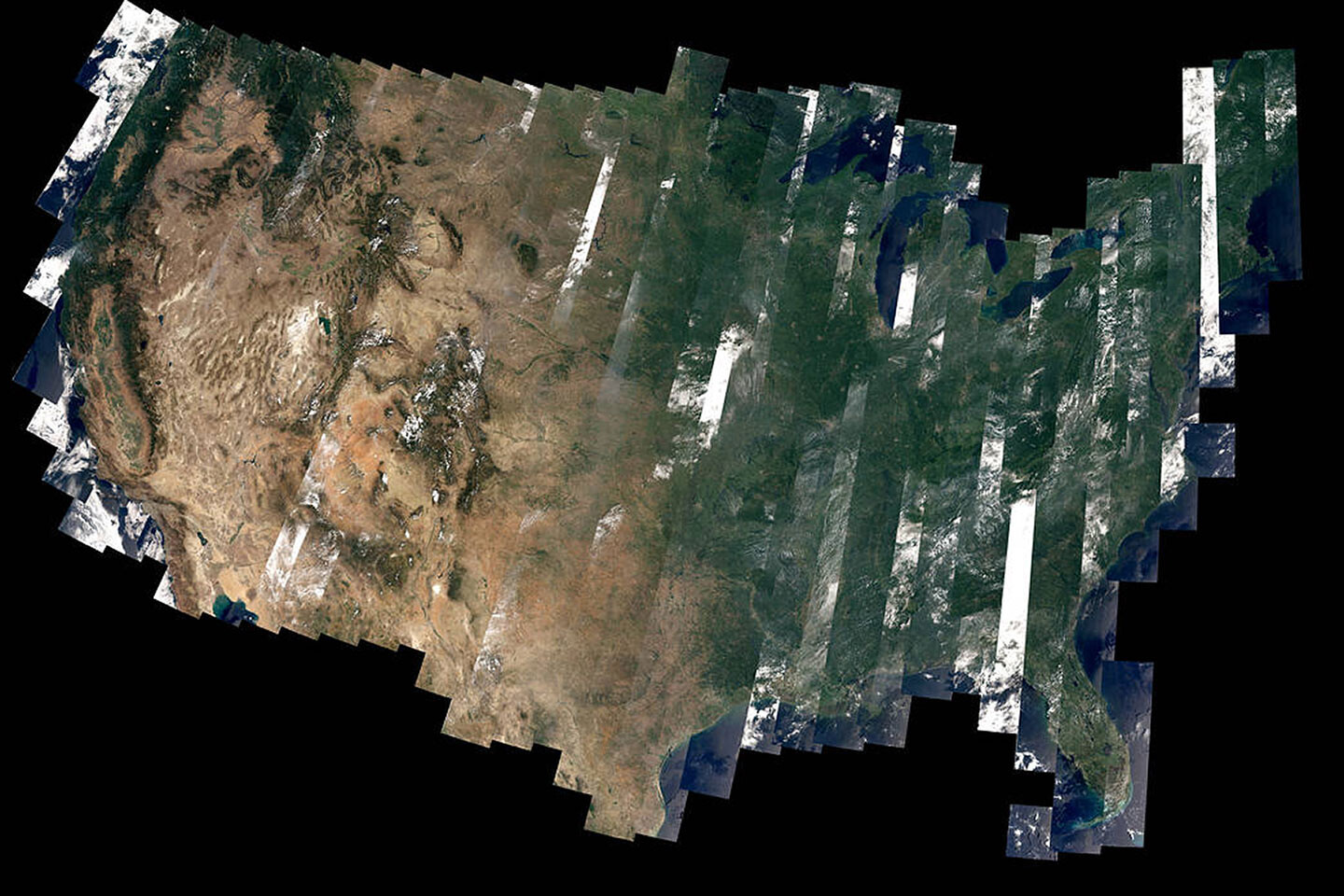
One of the first complete views of the United States from Landsat 8 is an example of how scientists are testing Landsat 8 data. The strips in the image above are a result of the way Landsat 8 operates. Like its predecessors, Landsat 8 collects data in 185-kilometer (115-mile) wide strips called swaths or paths. Each orbit follows a predetermined ground track so that the same path is imaged each time an orbit is repeated. It takes 233 paths and 16 days to cover all of the land on Earth. Photo: NASA/David Roy
These questions require us to focus not on how intelligent machines represent knowledge as an aggregation of data-facts; we must also embark on a materialist inquiry into the technical entrenching of thinking in artificial neural networks. One could argue that since World War II, algorithmic means of thinking should also be regarded as a mode of reasoning. It is true to say that most machine-learning algorithms, such as Netflix algorithms, actually focus on the specific use of data through a heuristic analysis of data correlations, statistically matching and thus predicting your data categories of preference, according to what you already may know. However, deep-learning algorithms—as a means of thinking how to think—involve not only the predictive analysis of content and the microtargeting of data use, but also define a tendency in artificial intelligence to abstract modalities of learning about infinite varieties of contextual content. These infinite varieties are not only derived from the algorithmic recording of the human use of data according to frequencies, contexts, and content, but also include the meta-elaboration of how algorithms have learned about these usages.
For instance, unlike recommendation algorithms, the RankBrain interpreter algorithms that support Google Ranking are not limited to making suggestions. Rather, they activate a meta-relational level of inference—i.e., the algorithm seeks an explanation for unknown signs in order to derive information—through the hypothetical conjectures of data involving algorithmic searches of indeterminate words, events, or things for which one may not have the exact search terms. As opposed to the heuristic analysis of data correlations among distinct sets, these interpreter algorithms do not just prove, verify, or validate hypothesis, but must first of all elaborate hypothetical reasoning based on what other algorithms have already searched, in order to determine the possible meaning of the missing information in the query. These deep-learning algorithms work by searching elements of surprise—that is, unthought information—which can only occur if the system is apt to preserve, rather than eliminate as errors, micro-levels of randomness that become manifested across volumes of data. In other words, this meta-digital form of automated cognition is geared not toward correcting errors or eliminating randomness; instead, it is indifferent to the entropic noise of increasing data volumes insofar as this noise is precisely part of the learning process, and for this reason experimental hypothesis-making must preserve indeterminacy so that it can bind information to surprise. While one can assume that this inclusion of indeterminacy—or irrationality or nonconscious activity—within the computational process is but another manifestation of the ultimate techno-mastery of reality, it is important here to reiterate instead that randomness is at the core of algorithmic mediation, and as such it opens up the question of epistemological mastery to the centrality of contingency within the functioning of any rational system. This results not in a necessary malfunction of a system—i.e., the line of flight of the glitch or the breakdown of order—but instead in its hyperrational (or sur-rational, to use Bachelard’s term) articulation of the real, the unknown, the incomputable, in terms of technical mediations, automated actualizations, and machine becomings of the real in their manifest artificial forms.
Instead of establishing general results from particular conceptual associations derived from the frequent use of particular content by humans and machines, the inclusion of indeterminacy in machine learning concerns the parallelism of temporalities of learning and processing, which involves an elaboration of data that sidesteps a primary level of feedback response based on an already known result.
We know that RankBrain algorithms are also called signals, because they give page rank algorithms clues about content: they search for words on a page, links to pages, the locations of users, their browsing history, or check the domain registration, the duplication of content, etc. These signals were developed to support the core Page Ranking algorithm so that in can index new information content.14 By indexing information, RankBrain aims to interpret user searches by inferring the content of words, phrases, and sentences through the application of synonyms or stemming lists. Here, the channeling of algorithmic searches towards already planned results is overlapped by an algorithmic hypothesis that is exposed to the indeterminacy of outputs and the random quantities of information held in the hidden layers of neural networks. For instance, indexing involves information attached to long-tail queries, which are used to add more context-specificity to the content of searches. Instead of matching concepts, RankBrain algorithms rely on the indeterminacy of results.
Indeterminacy has become part of the meta-digital synthesis of ratio and logos. It is an active element of this artificial form of knowing how, where the preservation of unknowns subtends a hypothetical inferencing of meaning: the meta-digital indifference to truth and fact may rather involve an opportunity to readdress instrumental knowledge in terms of hypothesis generation, working from within and throughout the means of thinking. In the age of post-truth politics, indeterminacy within machine learning defines not an external contingency disturbing an otherwise stable governance of information. Instead, the correlation of the “new brutality” of fake and alternative news with the contemporary form of automation involves a granular structuring of unknowns, pushing automated cognition beyond knowledge-based systems.15 Indeterminacy is therefore intrinsic to the algorithmic generation of hypothesis and as such the technoscientific articulation of truths and facts can no longer be confined to recurring functions and executions of the already known. The correlation between post-truth politics and automated cognition therefore needs to be further explored, contested, and reinvented.
As Deleuze and Guattari remind us, if we simply react to the dominant determinations of our epoch, we condemn thinking to doxa.16 In particular, if the dominant political rules of lying and bullying are facilitated by a rampant neo-heuristic trust in algorithmic search, would a nonreactive critique necessarily place philosophy outside of information technology?
Deleuze and Guattari already argued that philosophy must directly confront this new kind of dogmatic image of thought formed by cybernetic communication, which constantly fuses the past and future, memory and hope, in the continuous circle of the present. To think outside the dominance of the present, however, does not require a return to eternal truth—to the metaphysics of true ideas—that must be reestablished against the false. If cybernetics coincides with the information network of communication exchange subtending the proliferation of opinions and generating consensus,17 philosophy must instead make an effort to create critical concepts that evacuate the presence of the present from the future image of thinking. But how do you do this?
Deleuze and Guattari’s critique of communications society is a critique of computer science, marketing, design, and advertising.18 Communication is here understood as an extension of doxa, a model of the recognition of truth, which endlessly reiterates what everyone knows, what a survey says, what the majority believes. For Deleuze and Guattari, communication has impoverished philosophy and has insinuated itself in the micro-movements of thinking by turning time in a chronological sequence of possibilities, a linear managing of time relying on what has already been imagined, known, or lived. In opposition to this, Deleuze and Guattari argue that the untimely must act on the present to give space to another time to come: a thought of the future.
In the interview “On the New Philosophers and a More General Problem,” Deleuze specifically laments the surrendering of philosophical thought to media.19 Here, writing and thinking are transformed into a commercial event, an exhibition, a promotion. Deleuze insists that philosophy must instead be occupied with the formation of problems and the creation of concepts. It is the untimely of thought and the nonphilosophy of philosophy that will enable the creation of a truly critical concept. But how do we overturn the presumed self-erasure of critical concepts that stand outside the technoscientific regime of communication? Can a truly critical concept survive the indifferent new brutality of our post-truth and post-fact world, driven as it is by automated thinking? Doesn’t this mistrust of technoscience ultimately prevent philosophy from becoming a conceptual enaction of a world to come? Why does philosophy continue to ignore thinking machines that create alien concepts, acting as if there were beyond the capacity of machines to do?
Although they are worlds apart, one could follow a thread in Heidegger and Deleuze that asserts a defense of philosophy against technoscience, against the poverty of thought in the age of automated thinking. If the Heideggerian un-concealing of truth ultimately contemplates an unreachable state delimited by the awareness of finitude (of Western metaphysics, and of Man), Deleuze’s vision of the unthought of philosophy rather tends towards a creative unfolding of potentialities, the construction of conceptual personae that resist and counter-actualize the doxa of the present.
And yet a strong mistrust of technoscience prevails here. In particular, the forms of instrumental reason embedded in cybernetic and computational communication continue to be identified here with control as governance. Similarly, the conception of the mean or instrument of governance—that is, information technology—is left as a black box that has no aims (it is in itself a mindless, nonconscious automata) unless these are politically orchestrated. While it is not possible to disentangle the political condition of truth and fact from the computational processing of data retrieval and transmission, it seems also self-delimiting to not account for a mode of thinking engendered from the instruments or means of thinking.
If, with cybernetics and computation, the instrument of calculation has become a learning machine that has internally challenged technoscience—the logic of deductive truth and inductive facts—it is also because this form of instrumentality has its own reasoning, whereby heuristic testing has shifted towards hypothesis generation, a mode of thinking that exceeds its mode of doing. One could then argue that by learning to learn how to think, this instrumental form of reasoning transcends the ontic condition in which it was inscribed by the modern project of philosophy.
Since instruments are already doing politics, one question to ask is how to reorient the brutality of instrumentality away from the senseless stirring of beliefs and desires, and towards a dynamic of reasoning that affords the re-articulation—rather than the elimination—of aims. One possibility for addressing the politics of machines is to work through a philosophy of another kind, starting not only from the unthought of thinking, but also from the inhumanness of instrumentality, an awareness of alienness within reasoning that could be the starting point for envisioning a techno-philosophy, the reprogramming of thinking through and with machines. If the antagonism between automation and philosophy is predicated on the instrumental use of thinking, techno-philosophy should instead suggest not an opposition, but a parallel articulation of philosophies of machines contributing to the reinvention of worlds, truths, and facts that exist and can change.
The new brutality of technopolitics could be then overturned by a techno-philosophy whose attempts at reinventing the sociality of instrumental reasoning can already be found across histories, cultures, and aesthetics that have reversed the metaphysics of presence. For instance, the New Brutalist architecture of the 1950s to the 1970s activated a program for technosocial living that aimed to abolish the sentimental attachment to the end of the “spiritual in Man” and transcend the norm of architectural expression through an uninhibited functionalism, expressing the crudity of structure and materials.20 Not only functionalism, but also and above all a-formalism and topology were part of the procedural activities that tried to surpass the contemplation of the end by reimagining the spatial experience of truths and facts. Here, all the media are parallel or leveled together, in order to connect their specific content in a-formal dimension—one that could work through the incompleteness of a total image of sociality. The adjunction of the material world of animate and inanimate media however preserves the dissembling complexity of these discrete parts that can then revise mediatic forms of truths and facts into multiple directions.
The architecture of New Brutalism turns instruments of thinking into concrete, mass-modular, interconnected blocks and self-contained individual cells, elevated above the local territory, united by streets in the sky or networks of corridors across discrete parts of buildings. Here, instrumental reasoning transforms the entropic dissolution of the postwar period, holding the concrete weight of the past in order to dissolve it into structural experiments of task-oriented functions and aesthetic transparency. If New Brutalism had a vision that facilitated a weaponization of information, it was not simply to advance information technology, but rather to propose modes of instrumental reasoning that work through entropy, randomness, or noise to reprogram codes and values, passages and bridges, contents and expressions of a united image of the social.
Martin Heidegger, “The End of Philosophy and the Task of Thinking,” trans. J. Stambaugh, in Martin Heidegger, Basic Writings, ed. David Farrell Krell (London: Routledge, 1993), 373–92.
Ibid., 376.
Ibid.
Ibid., 392.
Ibid.
See for instance Gilles Deleuze, “Thought and Cinema,” chap. 7, in Cinema II: The Time-Image (Oxford: Athlone Press, 2000). See also François Laruelle, “The Transcendental Computer: A Non-Philosophical Utopia,” trans. Taylor Adkins and Chris Eby, Speculative Heresy, August 26, 2013 →.
See Ronald R. Kline, The Cybernetic Moment: Or Why We Call Our Age The Information Age (Baltimore: Johns Hopkins University Press, 2015), 53.
Ibid., 56.
For example, an AI system observing an image of a face with one eye in shadow may initially only see one eye. But after detecting that a face is present, it can then infer that a second eye is probably present as well. In this case, the graph of concepts only includes two layers—a layer for eyes and a layer for faces—but the graph of computations includes 2n layers, if we refine our estimate of each concept given the other n times.
See Aylin Caliskan, Joanna J. Bryson, and Arvind Narayanan, “Semantics derived automatically from language corpora contain human-like biases,” Science 356, no. 6334 (2017): 183–86.
Katherine N. Hayles, “Cognition Everywhere: The Rise of the Cognitive Nonconscious and the Costs of Consciousness,” New Literary History 45, no. 2 (2014).
Lorraine Daston, “The Rule of Rules,” lecture, Wissenschaftskolleg Berlin, November 21, 2010.
Katherine N. Hayles, How We Think: Digital Media and Contemporary Technogenesis (Chicago: University of Chicago Press, 2012).
Renée Ridgway, “From Page Rank to Rank Brain,” 2017 →.
See Rosi Braidotti, Timotheus Vermeulen, Julieta Aranda, Brian Kuan Wood, Stephen Squibb, and Anton Vidokle, “Editorial: The New Brutality,” e-flux journal 83 (June 2017) →.
Gille Deleuze and Félix Guattari, What Is Philosophy? trans. Hugh Tomlinson and Graham Burchell (New York: Columbia University Press, 1994), 99.
Ibid.
Ibid., 10.
Gille Deleuze, “On the New Philosophers and a More General Problem,” Discourse: Journal for Theoretical Studies in Media and Culture 20, no. 3 (1998).
Reyner Banham, The New Brutalism: Ethic or Aesthetic? (London: Architectural Press, 1966).